IBM's watsonx.data and Amazon's Kiro Lead the Charge in Revolutionizing Agentic AI and Automation in Enterprises
May 07, 2025
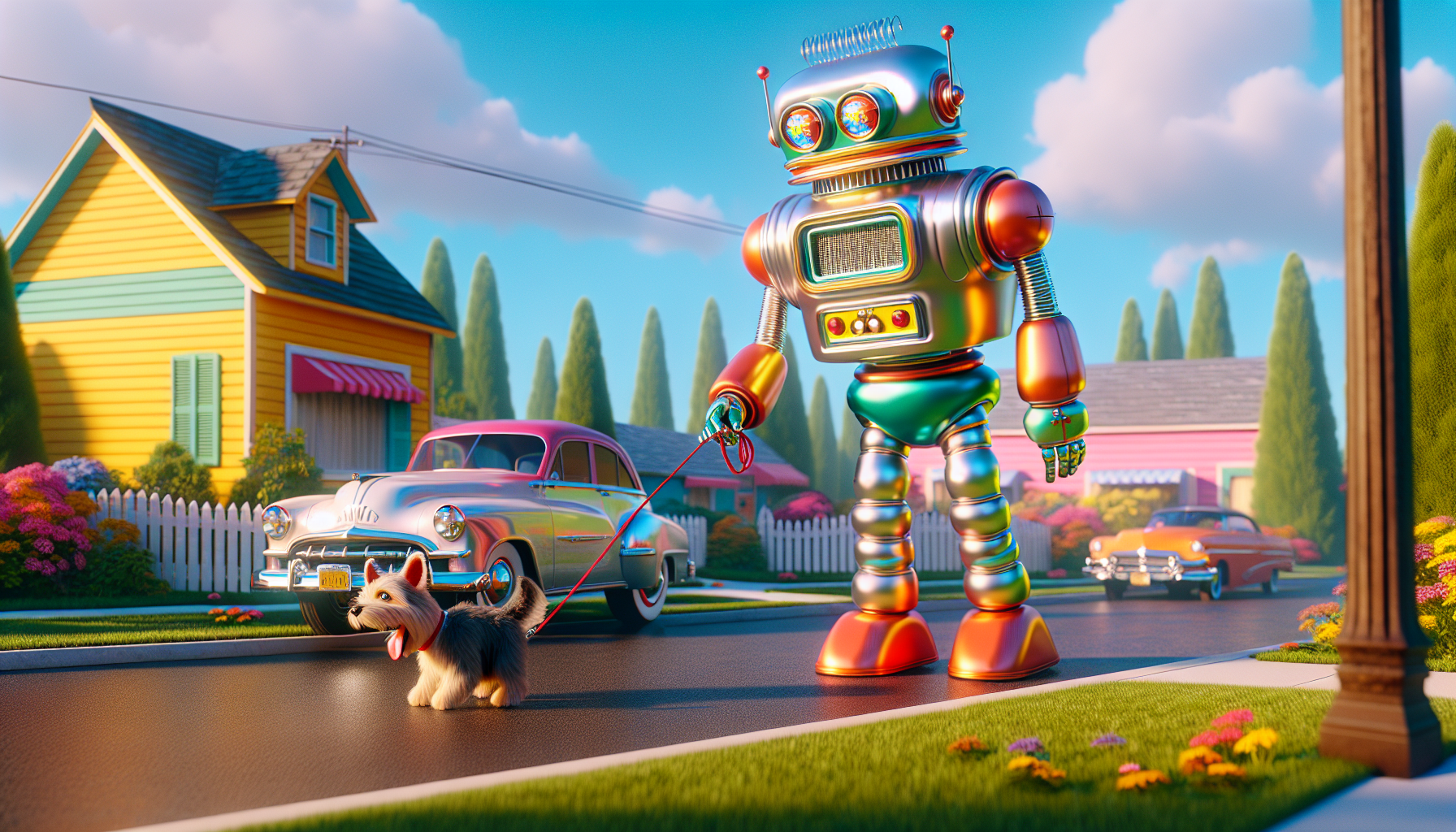
IBM's watsonx.data could simplify agentic AI-related data issues
IBM has enhanced its watsonx.data platform to tackle data challenges associated with agentic AI, offering new capabilities for better data management, analysis, and governance. The company emphasized that the primary obstacle for enterprises in harnessing the full potential of agentic and generative AI lies in unstructured data rather than the costs of inference. Edward Calvesbert, VP of product management for watsonx, highlighted that many businesses are overlooking foundational data issues by concentrating only on the generative AI application layer, which undermines the success of AI agents and initiatives. (Source)
Amazon is working on a secret project called 'Kiro,' a new tool that uses AI agents to streamline software coding
Amazon Web Services (AWS) is developing a new AI-powered coding tool, codenamed Kiro, aimed at revolutionizing software development by generating code nearly in real-time through the use of AI agents. Kiro, which can be integrated with both first-party and third-party AI agents, is designed to increase developer productivity by auto-generating technical documents, flagging issues, and optimizing code. This contrasts with current AI coding tools that Amazon critiques for their code-centric limitations. The rapid growth of AI coding assistants, highlighted by tools like Cursor and Replit, is reshaping the industry, prompting Amazon to address its previous challenges with its current AI tool called Amazon Q by exploring innovative new software development approaches. Amazon's initiative reflects a broader industry trend, with giants like Google and Microsoft leveraging AI for significant portions of their code writing, indicating a broader shift towards AI-driven coding solutions. (Source)
Chatbots out, AI agents in: What the future of customer service looks like
Keith Shaw discusses his frustrations with outdated customer service technologies with Glenn Nethercutt, CTO at Genesys. They address common issues like unhelpful IVR systems that trap users in automated loops, the redundancy of having to repeatedly provide personal information to different agents, and chatbots that simply follow rigid flowcharts without resolving issues. Glenn offers insights into how generative AI is beginning to address these challenges by improving system connectivity and enhancing chatbot capabilities, potentially offering more intelligent and seamless customer service experiences. (Source)
Real-world use cases for agentic AI
Agentic AI, an advanced stage of generative AI involving autonomous actions and complex workflows, is rapidly gaining traction in industries despite its complexity. Surveys by Cloudera and SnapLogic reveal a high adoption rate and future investment plans, with Gartner predicting AI agents will significantly influence enterprise functions by 2029. Notably, in software development, AI agents like GitHub Copilot and Amazon's Q Developer enhance productivity beyond traditional chatbots by autonomously managing entire projects. Companies such as Thomson Reuters and Bosch are integrating agentic AI in their processes, utilizing it for tasks like customer service and document analysis. Meanwhile, platforms like Google Vertex AI and Microsoft's Copilot Studio are democratizing AI agent creation, although some skepticism and challenges, particularly regarding widespread standardization, remain in this emerging technology. (Source)
Beyond Prompt-And-Pray: Why Structured Automation Is The Future Of Enterprise AI
Alan Nichol, Co-founder and CTO of Rasa, emphasizes the limitations of fully agentic AI in enterprise applications, highlighting issues such as unpredictable behavior, escalating costs, and security concerns. Nichol identifies three architectural approaches for integrating large language models (LLMs) into enterprise systems: the Full Agentic Model, which is flexible but unreliable; the Hybrid Model, which balances LLMs with rule-based systems; and Structured Automation, which separates conversational tasks from business logic to ensure reliability. Structured Automation has proven to be the most effective in reducing costs and improving execution consistency. As enterprises move towards production-grade AI implementations, the focus shifts to building reliable, efficient, and scalable systems by leveraging LLMs for language tasks while using deterministic workflows for executing business logic, thereby addressing the challenges observed during the "prompt-and-pray" era of AI deployment. (Source)